Revolutionizing Patient Care: Leveraging Machine Learning and AI to Predict Surgery Outcomes
This is an AArete Healthcare Provider insight
Patient healthcare is undergoing a revolution with health systems, research enterprises, government agencies, and other constituents of the healthcare ecosystem expending significant resources on predicting disease, providing preventative care, and developing precision medicine personalized to the patient.
Driving this revolution are new methods to collect, process, and analyze patient care data that span multiple variables and longitudinal studies. Advanced machine learning techniques, artificial intelligence, and deep learning are transforming research studies by handling vast amounts of real-world data, integrating both structured and unstructured sources, and employing sophisticated algorithms to uncover critical insights that drive clinical decisions.
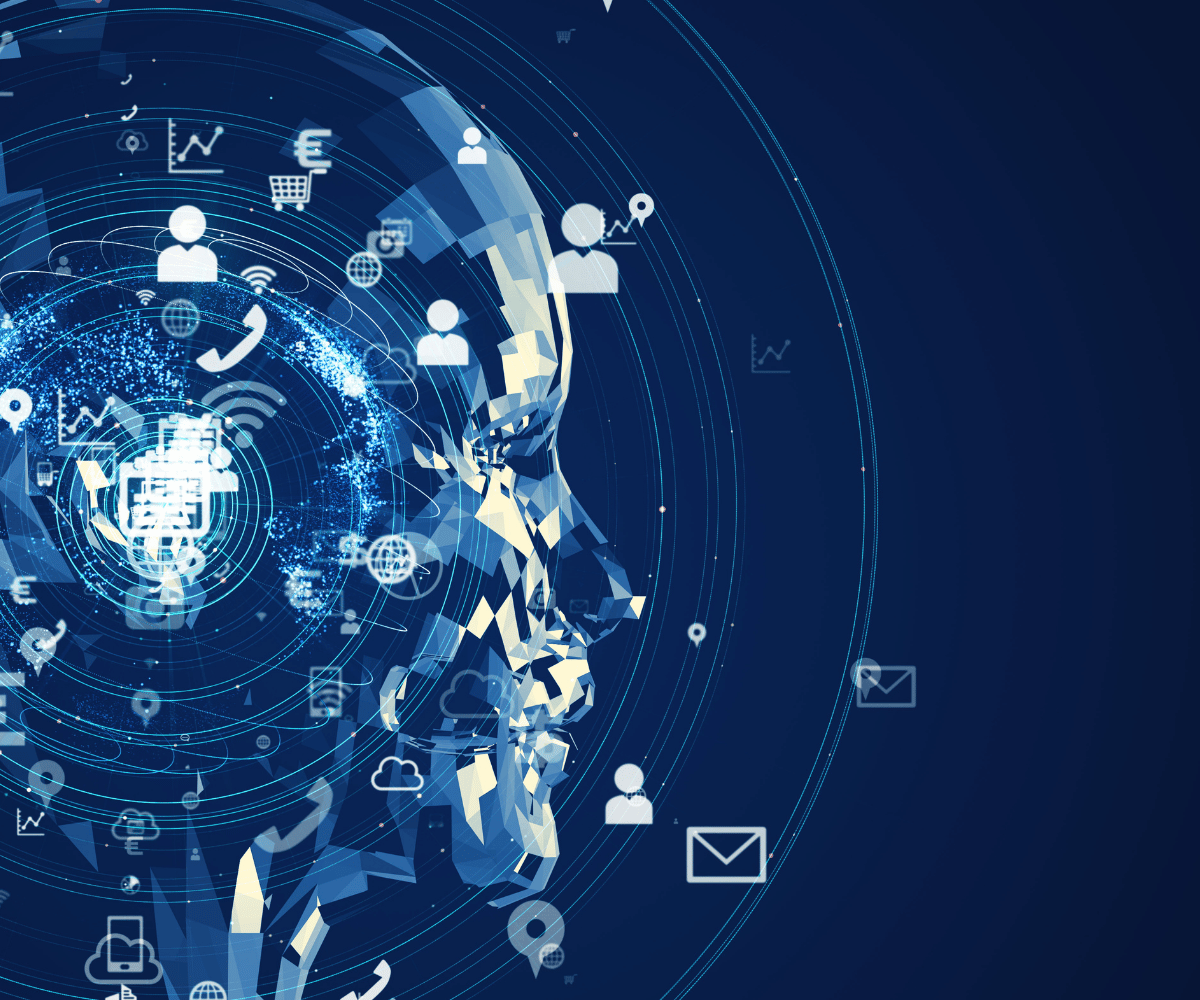
AArete’s team of data scientists recently partnered with a leading research and healthcare delivery institution to develop ML and AI-based models for the prediction of post-surgery arterial thrombotic — blood clotting — events incorporating results from Thromboelastography with platelet mapping (TEG-PM) provides comprehensive coagulation profiles that may enhance prediction accuracy. By leveraging this model, the care delivery can compute the probability of a thrombotic event following lower extremity revascularization for different time periods, and understand the impact of critical variables that may affect outcomes.
AArete is proud to be at the forefront of implementing practical and impactful AI that combines our deep expertise in healthcare with advanced data science skills.
The Research Initiative
Peripheral revascularization procedures are critical in restoring blood flow to arteries affected by occlusive diseases. However, despite their efficacy, patients undergoing these procedures face the risk of arterial thrombotic events post-operation, which can lead to severe complications and even mortality. Led by the associate professor of surgery and vascular surgeon and funded by the National Institutes of Health (NIH), the study’s primary objective is to advance our understanding of post-procedural arterial thrombotic events and develop predictive models for accurate identification.
AArete’s clinical and technical experts collaborated with the research team to prepare and shape extensive clinical data and built the model iteratively to derive insights related to demographics, procedural details, and post-operative outcomes. The resultant predictive model has the potential to improve clinical outcomes via timely interventions and precision medicine.
Methodology and Insights
Our research methodology combines meticulous patient data analysis with advanced machine learning algorithms to identify and prioritize key predictors of arterial thrombotic events following peripheral revascularization.
By following the lifecycle of a data science model, we supported data collection, preprocessing, and model development. Below, see the highlights of our approach:
- Data Collection: We compiled a comprehensive dataset integrating baseline patient demographics, medical history, procedural details, and biomarkers. These characteristics were merged with Thromboelastography (TEG) values, which measure blood clotting ability and were recorded at different time points. Additionally, synthetic data was integrated to enhance the dataset while maintaining data integrity.
- Data Preprocessing: Advanced imputation techniques were implemented to address missing data and bolster the dataset’s reliability. This process reduced biases related to data scale and format, resulting in more accurate model training.
- Model Development: Given the limited data, we applied techniques like SMOTE and other synthetic data methods to improve accuracy while maintaining sensitivity. Machine learning models explored included Logistic Regression, Decision Trees, Gradient Boosting, Support Vector Machines, Gaussian Naive Bayes, and k-nearest neighbors. Each model was rigorously trained and evaluated to determine the best fit for the task.
Adhering to these methodological standards guides AArete in producing scientifically sound insights that positively influence patient care strategies.
Expected Impact
The development and deployment of predictive models hold immense promise for enhancing patient care. These tools empower clinicians to identify high-risk patients, facilitate early targeted interventions, and develop new precision medicines.
How Can AArete Help?
AArete’s Digital & Technology Services group continues to support cutting-edge research using our unique combination of technical, clinical, and health economics expertise. Through partnerships with distinguished institutions and their research teams, we are implementing practical ML and AI techniques that drive health analytics and impactful research.
Connect With AArete
To learn more about this and other projects and explore how we can support your research initiatives, please complete this form and we will connect you with one of our experts.
Learn more about AArete’s Digital & Technology solutions